Abstract
INTRODUCTION
The identification of population-level healthcare needs using hospital electronic medical records (EMRs) is a promising approach for the evaluation and development of tailored healthcare services. Population segmentation based on healthcare needs may be possible using information on health and social service needs from EMRs. However, it is currently unknown if EMRs from restructured hospitals in Singapore provide information of sufficient quality for this purpose. We compared the inter-rater reliability between a population segment that was assigned prospectively and one that was assigned retrospectively based on EMR review.
METHODS
200 non-critical patients aged ≥ 55 years were prospectively evaluated by clinicians for their healthcare needs in the emergency department at Singapore General Hospital, Singapore. Trained clinician raters with no prior knowledge of these patients subsequently accessed the EMR up to the prospective rating date. A similar healthcare needs evaluation was conducted using the EMR. The inter-rater reliability between the two rating sets was evaluated using Cohen’s Kappa and the incidence of missing information was tabulated.
RESULTS
The inter-rater reliability for the medical ‘global impression’ rating was 0.37 for doctors and 0.35 for nurses. The inter-rater reliability for the same variable, retrospectively rated by two doctors, was 0.75. Variables with a higher incidence of missing EMR information such as ‘social support in case of need’ and ‘patient activation’ had poorer inter-rater reliability.
CONCLUSION
Pre-existing EMR systems may not capture sufficient information for reliable determination of healthcare needs. Thus, we should consider integrating policy-relevant healthcare need variables into EMRs.
INTRODUCTION
Singapore is ageing at an unprecedented rate. The proportion of the Singapore population aged 65 years and above will increase from 8.4% in 2005 to 18.7% in 2030.(1) In this era of increasing healthcare system burden, the development of tailored packages of services for distinct segments based on population needs holds significant potential for facilitating cost-effective, value-based and patient-centred care.(2-4) It is important to tailor healthcare services to healthcare needs, given that having insufficient services leads to unmet needs and worse clinical outcomes, while excessive or redundant services likely increase cost without improving health.(5-7)
There are two possible methods to efficiently obtain information on population-level healthcare needs. The first entails prospective collection of healthcare needs information using meso-level information on patient healthcare needs. This refers to, for example, ‘whether patient has a functional deficit’, as opposed to micro-level information detailing whether it is a deficit in ambulation, dressing or self-feeding. The Simple Segmentation Tool (SST) is one such instrument that can be used by clinicians to capture meso-level information; when aggregated, a snapshot of population-level healthcare needs is obtained. Research that formed the basis of the SST advocated healthcare needs-based population segmentation(3) and the inclusion of variables that were both predictive of future healthcare utilisation and informative for planning services at transitional points of care, such as physical function(8) and social support level.(9) The SST has been validated in terms of inter-rater reliability, as well as convergent and predictive validity in an outpatient setting. At the time of writing, the SST was not yet publicly available.
The second method entails retrospective determination of population healthcare needs information using the pre-existing electronic medical records (EMRs). Compared to the prospective method, the EMR system allows the pooling of large patient datasets in a less resource-intensive way, with a relatively high degree of clinical detail. It is thus a promising resource to inform policy decisions about the health services required and identify potential areas for improving healthcare integration.(10)
Nonetheless, EMRs may not always capture information in a reliable or accurate manner.(11) This could be due to variations found in clinician data entry, as well as the design of the EMR system, which has minimal data entry fields in order to avoid unduly burdening clinicians who perform data entry. At present, it is not known if EMRs from restructured hospitals in Singapore contain healthcare needs information of sufficient quality to facilitate meso-level healthcare needs-based segmentation. Hence, we aimed to evaluate the reliability of the EMRs by determining the inter-rater reliability of a brief patient healthcare needs identification instrument between clinicians who utilise the instrument in the clinic and those who utilise it based only on the EMRs. Secondarily, we aimed to determine the degree of missing information for selected healthcare need variables in the EMRs. We hypothesised that clinicians can reliably utilise EMRs to retrospectively identify healthcare needs information, and that poor reliability is due to a high degree of missing information.
METHODS
This retrospective study utilised a patient dataset containing SST ratings made prospectively in the emergency department, Singapore General Hospital, Singapore. The SST is a brief clinician-administered instrument developed in Singapore that segments patient populations into mutually exclusive health and health-related social service need segments (Appendix). It is designed for use in an outpatient setting, and clinicians trained in its use are expected to first assess a patient as part of their routine clinical assessment before using the instrument to categorise the patient.
The six medical ‘global impressions of patient’ of the SST were adapted from the original eight based on the ‘Bridges to Health’ model(3) in order to better suit our evaluation of an elderly population. Patients are classified into one of six health categories that best characterises their most salient clinical needs in the medium to long term (months to years), namely: (a) healthy; (b) chronic conditions and asymptomatic; (c) chronic conditions and stable; (d) long course of decline; (e) limited reserve and serious exacerbations; and (f) short period of decline before dying. All patients were only assigned to one category at any point in time. Although the SST version utilised in this study (Appendix) considers ‘Acutely ill but curable’ to be a global impression of patient category, it is analysed as a complicating factor, as it is a transient patient feature that can coexist with a patient’s baseline state (represented by the global impression of patient rating).
The ‘complicating factors’ section of the SST was designed to measure the degree of need in nine different healthcare-relevant characteristics: (a) functional assessment; (b) social support in case of need; (c) hospital admissions in the last six months; (d) disruptive behavioural issues; (e) polypharmacy; (f) organisation of care; (g) activation in the patient’s own care; (h) skilled nursing-type task needs; and (i) acutely ill but with curable conditions.
While primarily designed to capture policy-relevant information, the SST can also be used as a triaging tool for the identification of potentially complex patients who require more detailed evaluation. Depending on the highest level of complicating factor complexity identified in the patient, a complexity category can then be assigned (
Box 1
Complexity categories in the Simple Segmentation Tool:
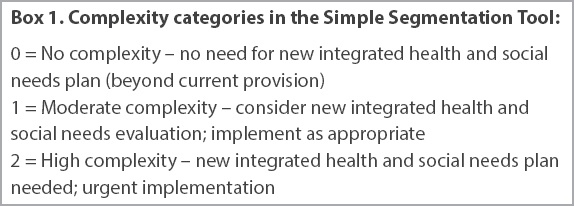
The dataset contained SST ratings from 200 non-critical patients aged ≥ 55 years. All patients were Singaporean citizens or permanent residents who provided consent for access to their EMRs. Patients were recruited in a contiguous manner. The prospective rater group comprised of doctors and nurses who observed patients’ interactions with their care provider in the emergency department clinic and reviewed the respective EMRs, then completed the SST for patients at the point of recruitment into the study.
Two medical doctors and one research nurse who had no knowledge of the patients’ prior SST ratings were provided with standardised training on the utilisation method of the SST and test cases to familiarise them with the EMRs for purposes of retrospective SST rating. During training, raters were provided with an algorithm (
Fig. 1
Flowchart shows algorithm to facilitate retrospective Simple Segmentation Tool global impression rating for all categories except Category II (acutely ill but curable).
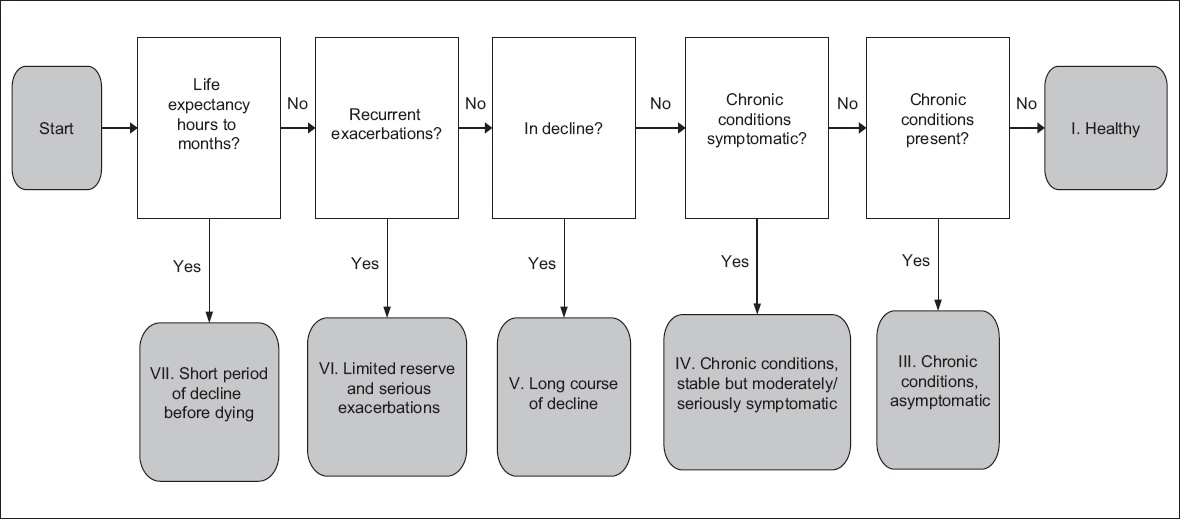
Table I
Reference table for rating retrospective complicating factors in the Simple Segmentation Tool.
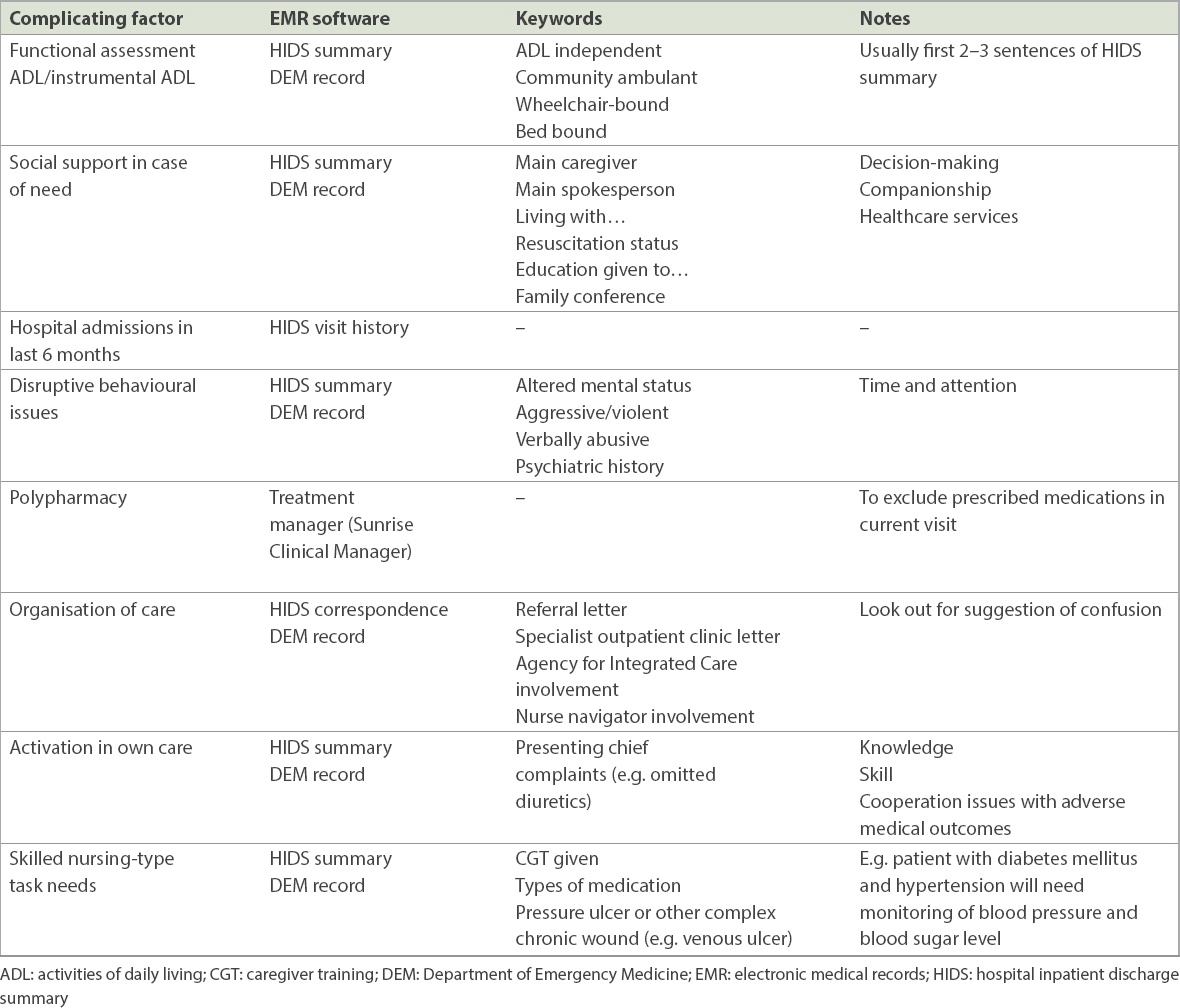
All raters were requested to review patient records only up to the time of the emergency department notes during which the prospective SST ratings were made. This was to reduce the risk of bias in retrospective ratings, and ensure that both retrospective and prospective SST rating were done during a similar time point. There was no limit to the earliest possible record that could be reviewed. Raters were allowed to review all data fields in the EMRs that fit within the stipulated time frame.
Retrospective SST raters independently reviewed the records using Singapore General Hospital’s Allscripts Sunrise Clinical Manager EMR system. While raters had access to all features of the EMRs, the discharge summaries and emergency department notes were most relevant for obtaining the required healthcare needs information to rate the SST. If a particular piece of information could not be found, raters were required to rate using their best guess and then mark on the SST instrument that the information was missing. The frequency of missing information was then tabulated for all SST data variables.
A sample size calculation was done based on the primary aim of determining the inter-rater reliability of prospective rating versus retrospective rating of the SST global impression rating. We found that a sample size of 139 subjects had 85% power to detect a true Kappa value of 0.60 with a significance level of 0.05.
Retrospective SST ratings by the medical doctors were compared with prospective ratings by the reference physician using Cohen’s Kappa coefficient. The inter-rater reliability of SST global impression ratings between the two retrospectively rating doctors was also determined. Meanwhile, the retrospective ratings by the research nurse were compared with the prospective ratings by other research nurses using Cohen’s Kappa. This study was approved by the SingHealth Centralised Institutional Review Board (CIRB/2016/2005).
RESULTS
Out of 200 patients, 60 patients were reviewed by both retrospective doctors, while 70 were only reviewed by the first retrospective doctor and another 70 were only reviewed by the second retrospective doctor. Thus, retrospective doctors reviewed a total of 130 patient records each, while the research nurse and prospectively rating reference physician reviewed all 200 records. Among the 140 patient records included in inter-rater reliability analysis between prospective and retrospective doctors, 60 records were reviewed by all three retrospective raters. 60 out of the initial 200 case records were excluded from the study, as these patients either decided to withhold consent for access to their EMRs or were utilised as teaching cases by the prospectively rating clinicians and were thus not independently rated. Patients were distributed according to the SST’s global impression and complicating factor categories, as rated by the reference physician (i.e. one of the prospectively rating clinicians), whose rating was taken to be the gold standard. Most patients were of low medical severity and were classified in the ‘chronic, asymptomatic’ category (
Table II
Distribution of patients according to reference physician’s global impression rating.
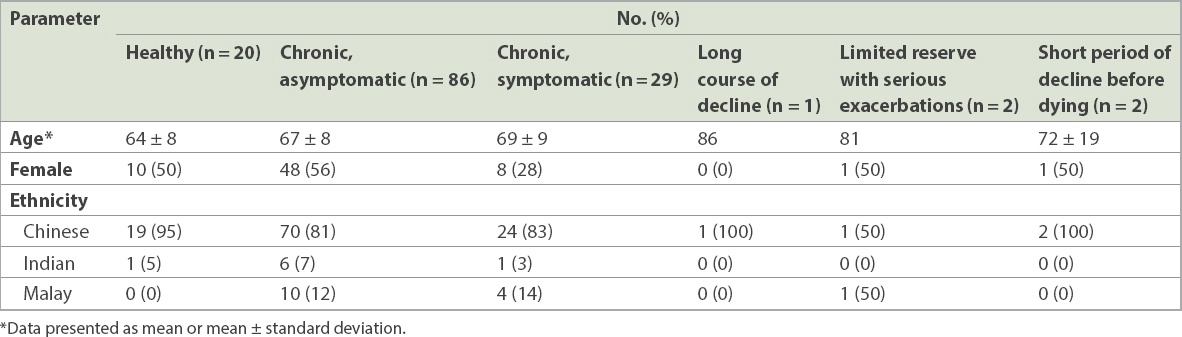
Table III
Distribution of patients according to reference physician’s complicating factor rating.

Data variables with less missing information such as past admissions, polypharmacy and global impression were found to have higher inter-rater reliability scores (
Table IV
Missing information and inter-rater reliability results, based on Simple Segmentation Tool data variables.
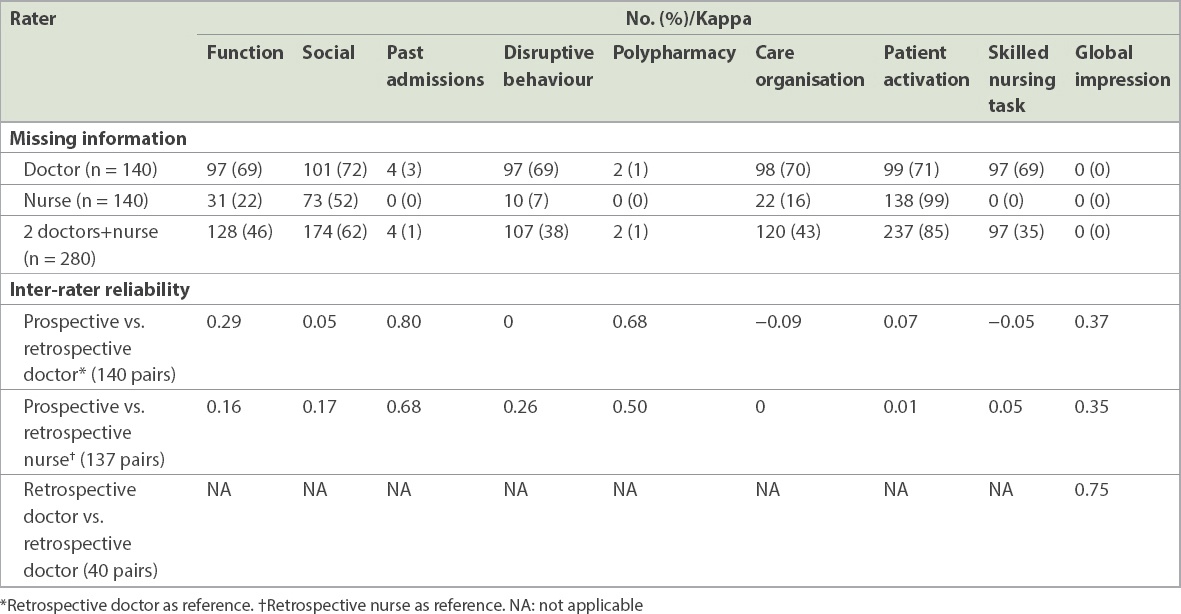
In terms of inter-rater reliability for SST global impression between prospective and retrospective ratings, the Cohen’s Kappa score was 0.37 and 0.35 for doctors and nurses, respectively. Although these fell short of a Cohen’s Kappa score of 0.6, which was set as the threshold of sufficient reliability in this study, the inter-rater reliability between the two retrospective doctors was significantly better, with a Cohen’s Kappa score of 0.75. This suggests that poor comparability between prospective and retrospective ratings is due to missing information within the EMRs, and not that the process of retrospective rating itself was inherently unreliable.
DISCUSSION
Moving forward, the EMR system has immense potential for patient stratification using real-time big data analytics. It is imperative that important variables are routinely captured as part of a holistic biopsychosocial approach to patient assessment. For instance, physical function,(12,13) social support(14-18) and patient activation(19) are well-known predictors of re-admission risk and can be used to identify care needs that require intervention. A comprehensive literature review is needed to locate variables with discriminatory and predictive value that can be included in the EMR.
SST variables with poor inter-rater reliability scores are important population healthcare need markers that can facilitate the planning and development of health service interventions. In order to improve the process of information capture by clinicians into the EMRs, one option would simply be to include these variables in the EMR system. This would improve data reliability for population-level health service policy decisions, while potentially reducing the amount of time needed for clinicians to input data into subjective data fields. Specific SST healthcare need variables with poor reliability that could benefit from such an intervention include physical function, social support in case of need, disruptive behavioural issues, care organisation, patient activation, skilled nursing task needs and global impression.
To the best of our knowledge, this is the first study in Singapore that examines the quality of a restructured hospital’s EMRs for purposes of retrospective healthcare need identification. The strengths of this study include its evaluation of reliability in retrospective rating for both medical doctors and nurses, as well as the characterisation of missing healthcare need information for key variables, which could facilitate targeted improvement of the EMR system through the addition of objective data fields.
One possible limitation of this study is that the emergency department EMRs were written by doctors who were prospectively rating patients using the SST. Hence, the quality of their records may be slightly different from the conventional records of doctors who did not use the SST. Nonetheless, our retrospective clinician raters have given feedback that there were no perceptible differences between their patient records and those of other records seen in their usual line of work, and thus the plausible biasing effect of creating records with more complete information is likely to be minimal. If bias had occurred, it would have strengthened the inter-rater reliability between the prospective and retrospective raters, yet strong inter-rater reliability was not observed. Another possible limitation would be that the patients recruited in this study had relatively low medical and social healthcare need complexity. Future studies may benefit from recruitment at sites such as the hospital inpatient department or emergency department areas with higher triage urgency, where patients typically have more healthcare needs.
In conclusion, our results suggest that the clinician’s best guess is no substitute for objectively recorded information in the EMRs in terms of identifying healthcare needs. Policymakers may consider integrating important healthcare need data variables into the EMR system as routine data fields to improve data quality and reliability.