Abstract
INTRODUCTION
The Kidney Failure Risk Equation (KFRE) was developed to predict the risk of progression to end-stage kidney disease (ESKD). Although the KFRE has been validated in multinational cohorts, the Southeast Asian population was under-represented. This study aimed to validate the KFRE in a multi-ethnic Singapore chronic kidney disease (CKD) cohort.
METHODS
Stage 3–5 CKD patients referred to the renal medicine department at Singapore General Hospital in 2009 were included. The primary outcome (time to ESKD) was traced until 30 June 2017. The eight- and four-variable KFRE (non-North America) models using age, gender, estimated glomerular filtration rate, urine albumin-creatinine ratio, serum albumin, phosphate, bicarbonate and calcium were validated in our cohort. Cox regression, likelihood ratio (Χ2), adequacy index, Harrell’s C-index and calibration curves were calculated to assess the predictive performance, discrimination and calibration of these models on the cohort.
RESULTS
A total of 1,128 patients were included. During the study period, 252 (22.3%) patients reached ESKD at a median time to ESKD of 84.8 (range 0.1–104.7) months. Both the eight- and four-variable KFRE models showed excellent predictive performance and discrimination (eight-variable: C-index 0.872, 95% confidence interval [CI] 0.850–0.894, adequacy index 97.3%; four-variable: C-index 0.874, 95% CI 0.852–0.896, adequacy index 97.9%). There was no incremental improvement in the prediction ability of the eight-variable model over the four-variable model in this cohort.
CONCLUSION
The KFRE was validated in a multi-ethnic Singapore CKD cohort. This risk score may help to identify patients requiring early renal care.
INTRODUCTION
Chronic kidney disease (CKD) has a high prevalence worldwide, ranging from 7.2% to 13.4%.(1-4) According to the Global Burden of Disease Study, the age-standardised prevalence rate for all-cause CKD was 6,973 cases per 100,000 people in 2013.(5) In Singapore, the prevalence of CKD was reported to be 15.6% in 2007.(6) This is projected to increase to 24.3% by 2035.(7) CKD patients are at risk of progression to end-stage kidney disease (ESKD), cardiovascular events and mortality.(8) Kidney failure considerably reduces life expectancy, and the costs of dialysis and transplantation consume disproportionate amounts of healthcare budgets in all jurisdictions.(9)
Owing to the heterogeneity of kidney diseases and variability of progression of CKD, provision of appropriate care and education to patients remains a challenge. Accurate prediction of risk of CKD progression may aid patients’ understanding about their own conditions and improve their compliance to CKD therapy. Tangri et al proposed and validated a Kidney Failure Risk Equation (KFRE) model for progression of chronic kidney disease to ESKD in a Canadian population.(10) Their group subsequently validated the KFRE in multinational cohorts.(11) The KFRE is available through electronic applications (e.g.
METHODS
The study population was derived from patients who were referred to the Department of Renal Medicine at Singapore General Hospital (SGH), Singapore, in 2009. The period of review was from the date of the initial referral to the date of kidney failure or last data collection (30 June 2017). Patients aged > 21 years with CKD Stage 3–5 (estimated glomerular filtration rate [eGFR] < 60 mL/min/1.73 m2) at the time of the initial renal medicine referral were included. Participants were excluded from the study if they did not have recorded baseline eGFR or albuminuria levels, or if renal replacement therapy or kidney transplantation had already been initiated at the time of the initial renal medicine referral. Outcomes were ascertained through retrospective reviews of clinical records and clinical information from the SGH dialysis database for the duration of the study period. The research was approved by the SingHealth Centralised Institutional Review Board (reference no. 2016/2088).
The primary outcome measure was the time to ESKD, defined as the time from initial referral to the SGH renal medicine department to the development of ESKD. ESKD was defined as the initiation of dialysis or kidney transplantation.
The four-variable (age, gender, baseline eGFR and log urine albumin-creatinine ratio [ACR]) and eight-variable (age, gender, baseline eGFR, log urine ACR, serum albumin, serum phosphate, serum bicarbonate and serum calcium) non-North America KFRE models were validated in this study.(11) The variables were evaluated as continuous variables. They were obtained from the renal clinic records and were restricted to within 90 days of the initial referral to the department. eGFR was calculated using the Chronic Kidney Disease Epidemiology Collaboration (i.e. CKD-EPI) equation with serum creatinine standardised to isotope dilution mass spectrometry-traceable methods.(14) Albuminuria was represented as a log-transformed urine ACR owing to its skewed distribution in the original KFRE article.(10) Alternative measures of proteinuria (urine protein-creatinine ratio and 24-hour urine total protein) were converted to ACR using previously developed equations.(11) Moderately increased albuminuria was defined as urine albumin-creatinine ratio 30–299 mg/g, while severely increased albuminuria was defined as urine albumin-creatinine ratio ≥ 300 mg/g.(9) Serum albumin, serum phosphate and serum calcium were converted from SI units to their conventional units. Serum bicarbonate was converted from SI unit to mEq/L before being tested in the KFRE models.(11)
Univariable Cox regression analyses were performed to evaluate the effects of individual variables in the eight- and four-variable KFRE models on the risk of ESKD. The likelihood ratio chi-square test and adequacy index were used to assess the predictive performance of the four- and eight-variable KFRE models. For measurement of discrimination, Harrell’s C-index was calculated to evaluate the probability of concordance between the predicted and observed risk of kidney failure, with a C-index of 0.5 representing a random prediction and C-index of 1.0 representing a perfectly discriminating model. Calibration (i.e. the difference between observed and predicted risk) was examined by plotting the observed two-year and five-year probability of ESKD in this cohort and comparing it with the predicted risk using the (non-North America) KFRE.
Kaplan-Meier curves were drawn to illustrate the difference in time to ESKD among predetermined KFRE five-year risk groups of low risk (0%–4.99%), intermediate risk (5.00%–14.99%) and high risk (≥ 15.00%), as defined by the original KFRE article.(10) All statistical analyses were performed using R version 3.4.2 (R Foundation for Statistical Computing, Vienna, Austria). A two-sided p-value < 0.05 was considered statistically significant.
RESULTS
A total of 1,128 patients met the inclusion criteria. During the follow-up period, 252 (22.3%) patients progressed to ESKD, the primary endpoint. The median time to ESKD was 84.8 (range 0.1–104.7) months. 383 (34.0%) patients died before reaching the primary endpoint and were considered as censored, similar to the original KFRE article.(10)
Patient demographics, baseline clinical parameters and laboratory data are shown in
Table I
Baseline characteristics of the Singapore cohort (n = 1,128).
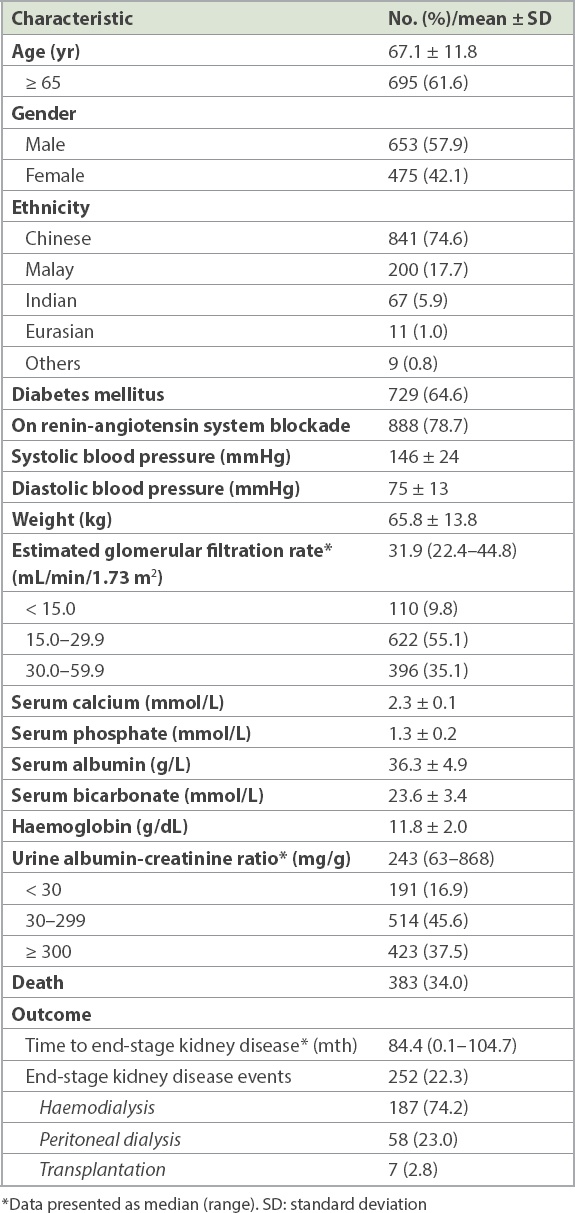
Univariable Cox regression showed that the higher the log urine ACR (hazard ratio [HR] 2.26, 95% confidence interval [CI] 2.03–2.52) and serum phosphate (HR 2.86, 95% CI 2.42–3.37), the higher the risk of developing ESKD, while the higher the age (HR 0.67, 95% CI 0.61–0.74), eGFR (HR 0.64, 95% CI 0.60–0.68), serum albumin (HR 0.45, 95% CI 0.39–0.51), serum bicarbonate (HR 0.88, 95% CI 0.85–0.91) and serum calcium (HR 0.28, 95% CI 0.22–0.35), the lower the risk of developing ESKD. Compared to female patients, male patients had a lower risk of developing ESKD (HR 0.73, 95% CI 0.57–0.93). Compared to Chinese patients, Malay patients had a higher risk of developing ESKD (HR 1.88, 95% CI 1.42–2.49), while there was no significant difference in the risk for Indian and Eurasian patients (
Table II
Univariable analysis of time to kidney failure.
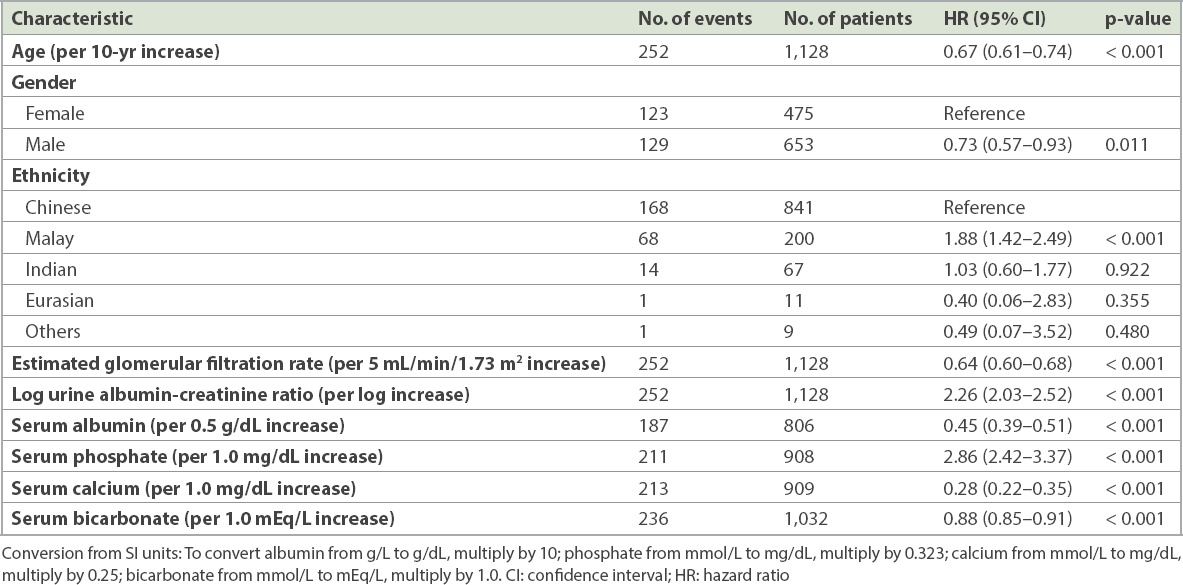
Overall, the KFRE models had excellent predictive performance and provided excellent discrimination, which were similar whether the four- or eight-variable KFRE models was used. The likelihood ratios for the four-variable and eight-variable KFRE models were 352.8 (p = 0.002) and 350.5 (p = 0.006), respectively, and the adequacy indexes of these two models were similar (four-variable 97.9% vs. eight-variable 97.3%). Cox regression showed that patients with a higher score for either model had a higher chance of developing ESKD (eight-variable model: C-index 0.872, 95% CI 0.850–0.894; four-variable model: C-index 0.874, 95% CI 0.852–0.896). The calibration curves of the eight- and four-variable models display the relationship between the predicted risk and the true event probabilities (
Fig. 1
Charts show calibration of the four-variable and eight-variable models in terms of two-year and five-year kidney failure rates.
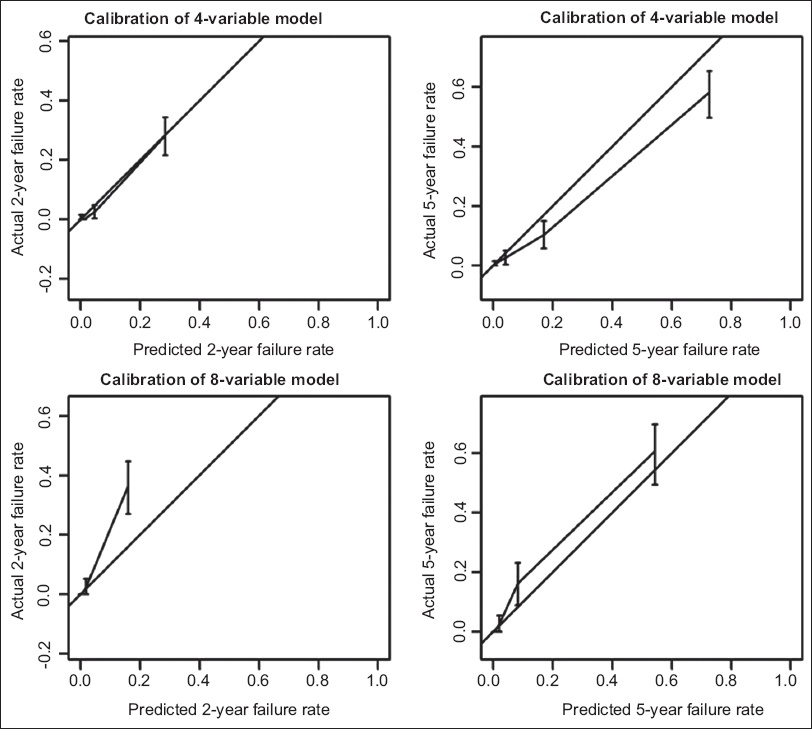
The Kaplan-Meier curves for estimated time to ESKD based on five-year risk grouping are shown in
Fig. 2
Kaplan-Meier curves show the estimated time to kidney failure (event) based on five-year risk using (a) an eight-variable model and (b) a four-variable model. The grey area denotes the 95% confidence interval of the event-free rate (low risk: 0%–4.99%, intermediate risk: 5.00%–14.99%, high risk: ≥ 15.00%).
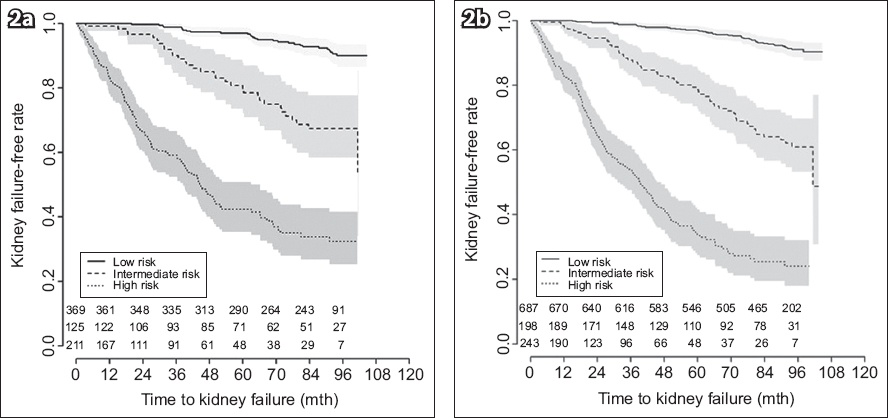
DISCUSSION
Our cohort was derived from new referrals at primary healthcare clinics across Singapore in 2009 who were followed up longitudinally. This cohort is reflective of the ethnic makeup of the general Singapore population.(15) We validated both the eight-variable and four-variable KFRE (non-North America) models for risk of progression of CKD to ESKD in a CKD cohort in Singapore. In our cohort, there was no incremental improvement in the predictive ability of the eight-variable model over the four-variable model. Based on the calibration curves, the actual and predicted five-year-risk ESKD rates for both models are well matched, with no further calibration required for our local cohort. In view of the good discrimination and calibration of the existing KFRE models for our local cohort, we did not proceed to revise the models. However, we did explore creating a new model by including local ethnicities as an additional variable into the original KFRE model. Nevertheless, this did not significantly improve its discrimination ability.
We followed the original KFRE article and censored the patients who had died before developing kidney failure during the study period. We agreed with the authors of the original KFRE article that predicting the risk of ESKD alone is important for decisions made by patients, physicians and healthcare systems.(10) In other medical conditions, risk prediction equations have led to better adherence to treatment guidelines and have encouraged individual decision-making.(16-18) Similarly, use of the KFRE can lead to similar outcomes in CKD management. The advantage of the KFRE is that it uses routinely checked parameters in CKD patients that can be easily obtained from patients’ electronic health records (EHR). The KFRE can be incorporated into the EHR to enhance its usability. Based on the risk calculated, physicians can advise and educate patients on dialysis planning, timely vascular access creation and pre-emptive kidney transplantation to reduce the number of emergent unplanned hospital admissions for acute initiation of renal replacement therapy. A risk prediction-based approach may also help health administrators to allocate appropriate resources according to the risks their populations face and deliver cost-effective care to CKD patients.(19)
In Canada, risk-based triage for referral to renal medicine using KFRE has been investigated.(20) The study suggested improved wait times and access to care for patients at highest risk of progression to ESKD, but the long-term outcomes of low-risk patients were unknown.(20) Our study shows a clear distinction in kidney-failure-free rates between the different risk groups, which suggests that we can use the four-variable KFRE five-year prediction model to risk stratify CKD Stage 3 and 4 patients. Low-risk (0%–4.99%) patients can be managed at the primary healthcare level, high-risk (≥ 15.00%) patients need to be referred to renal physicians, and intermediate-risk (5.00%–14.99%) patients can be referred as per the discretion of the individual primary healthcare physician, based on suspicion of glomerulonephritis, uncontrolled hyperkalaemia, severe uncontrolled hypertension, or further investigation or treatment that is available only in hospitals. Hospital renal physicians can use similar KFRE risk stratification to discharge patients who become low risk after treatment. This will allow better allocation of limited medical resources and right-siting of patient care. Future studies can investigate the risk of referral back to renal medicine after patients are discharged to primary healthcare based on their KFRE risk.
Our study has several limitations. As a retrospective cohort study, it has inherent disadvantages. Potential unknown confounders may be present in a retrospective database, and not all the relevant data may have been available for analysis. There may be selection bias, where patients who were referred to renal physicians were those at higher risk of progression. The cohort used to analyse the eight-variable model was smaller than that of the four-variable model owing to missing data; this may have also resulted in selection bias and affected model accuracy. The cause of death for patients who died during the study period was not universally collected; hence, there was a chance that some of the deaths were related to end-stage kidney failure. This limitation was minimised by reviewing the clinical records to ensure that all patients who were started on dialysis prior to death were recorded as ESKD cases. Another limitation is the possibility that baseline serum creatinine and eGFR levels were affected by potential acute kidney injury events during the period of data collection, resulting in inaccurate baseline eGFR readings. We also postulate that the underlying cause of CKD, which was not included as one of the variables in these models, may potentially affect the performance of the models, as different aetiologies follow different trajectories in the progression to end-stage kidney failure.(21) While it would be interesting to evaluate if this affects the models, data collection would be limited by both the accuracy of the diagnosis of the cause in a retrospective database and the wide variety of causes of CKD, especially with the numerous subcategories of glomerulonephritis.
Future studies can look at the use of a prediction model for CKD patients to improve clinical outcomes and healthcare cost.(22) Future novel biomarkers identified for CKD prognostication can be incorporated into the prediction models to further improve their accuracy. Refining prediction models to provide an approximate time to ESKD, rather than risk category, may help patients to better understand the severity of their illness and empower them to manage their condition better. For example, Tangri et al presented a dynamic prediction model for progression of CKD.(23) This may provide better predictions for progression of CKD than the current static model (i.e. KFRE model). Future prospective studies can attempt to validate and improve the dynamic prediction model. Studies that combine prediction models to look at both composite and distinct outcomes of ESKD, cardiovascular events and all-cause mortality will provide a better representation of the potential significant outcomes that patients may face, which would aid decision-making for patient care.
In conclusion, the KFRE, which uses routine laboratory tests for predicting the risk of progression of CKD to ESKD, is validated in a multi-ethnic CKD cohort in Singapore. This risk score may help to identify patients who require early renal physician care.
ACKNOWLEDGEMENT
The research team would like to acknowledge the Singapore General Hospital Health Services Research Unit and Integrated Health Information Systems (funded by the Singapore General Hospital Health Services Research – Oracle Business Intelligence Enterprise Edition Wave 3) for their assistance in data extraction, project management and statistical analyses.